I have seen data science projects stuck in the minimum viable product (MVP) phase for years.
That's not good and shows that something isn't right. Here are the top three reasons why this happens.
First of all, the execution of a Data Science project can be divided into the 4 steps Proof of Concept (PoC), Minimum Viable Product (MVP), Validation and Scaling.
Getting from one stage of your project to another requires a lot of work. Overall, a data science project needs work, money and people as input.
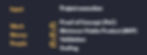
But if you get bogged down and if you don’t have the necessary resources, your project will fail after the MVP. Which means you won’t get to the next step, the validation.
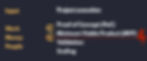
Another point where projects often fail is after the validation.
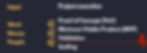
The worst case, however, is when you do a Proof of Concept but can’t go to the Minimum Viable Product because you need people but can’t get them.
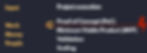
It is always possible that Data Science projects fail at one of these shown points, which is always very annoying but unfortunately happens.
Has one of your Data Science projects ever failed? If so, where and why? Write it down in the comments - I am looking forward to it!
- Become a Data Engineer: Click here! - My free 100+ pages Data Engineering Cookbook: Click here! - Follow us on LinkedIn: Click here! - Check out my YouTube channel: Click here!